Share this
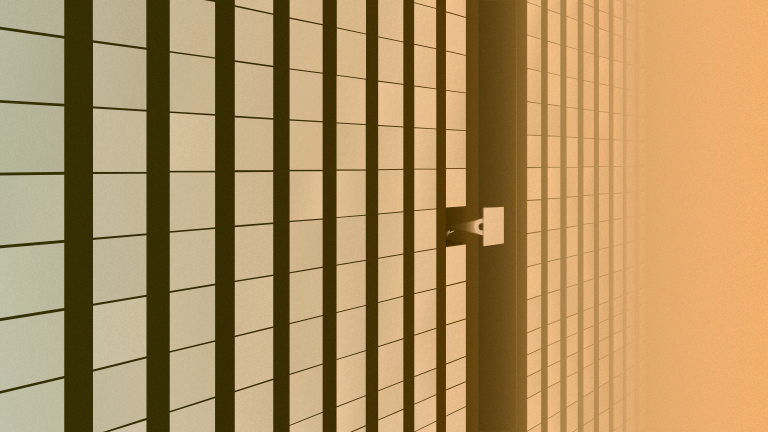
How AI-Inspired chess matches encourage innovation in healthcare privacy
by Matt Ford, Senior Machine Learning Engineer, Protenus on September 5, 2018
Artificial Intelligence (AI) is somewhat of a misnomer. It is indeed artificial, but it is not intelligent--at least not in the way a person is. The entire field of artificial intelligence grew out of research in the late 1940s and early 1950s that attempted to teach computers to play a game of chess. In 1997, this technology matured and advanced to the point where IBM’s Deep Blue supercomputer was able to beat chess Grandmaster Garry Kasparov. At a high level, computer chess engines rely on an intricate search engine coupled with a huge database of possible moves and games. Kasparov realized that this huge resource could be even more valuable when further coupled with human intelligence. This combination of human and artificial intelligence working together form a cyborg, or centaur, which represents the melding of true human intelligence with the power of advanced computational systems. These advances in technology have inspired the central role that AI plays in healthcare innovation. Specifically, in healthcare privacy, it serves as an extension of the team, better leveraging human expertise, and increasing their efficiency.
AI as an extension of the team
Scientists and engineers working hand-in-hand with computers is certainly nothing new. The power of a computer allows individuals to see potential solutions to a problem in a new light or allows a team of people to achieve more in less time. In my career before Protenus, I used a computer to help design new nanoscale materials for adsorbing gases for industrial uses. This work, which would have taken a highly trained chemist months to deploy, was able to be executed in just a few hours or days due to the use of available raw computing power in conjunction with human intelligence. In other words, this computing power served as an extension of my team. Similar processes take place in countless other scientific and engineering disciplines from drug discovery to air flow around F1 race cars to combustion and now healthcare and medicine.
Artificial intelligence is beginning to be used in medical research in promising ways. Certain types of machine learning systems--(convolutional) neural networks--have been trained to “understand” images. These systems have shown to be as good as or better than trained physicians at detecting tuberculosis in chest X-rays or picking out cancerous cells in a large microscopy image. Computers do not suffer from fatigue or oversight in the same way a human could after a long day of examining images, making the ability to run calculations endlessly a huge benefit. Going a step beyond, certain artificial intelligence programs can predict adverse cardiovascular events at a more accurate rate than a human using the American College of Cardiology/American Heart Association (ACC/AHA) guidelines. However, it must be understood that in every one of these cases, it was human expertise that showed the machines what they needed to learn. At Protenus, we are applying the math, science, and engineering that underlies these and similar AI applications to attack the problem of data privacy in healthcare.
Leveraging human expertise, not replacing it
Protenus provides health systems the ability to leverage the combination of human and artificial intelligence to accurately detect threats to patient privacy. A world-class team of data scientists and engineers built the AI-powered platform using the latest advances in technology to better detect instances of inappropriate access to patient data. The platform becomes a valuable extension of the privacy team, enabling them to only receive alerts that need their utmost attention so they can maintain focus on their top priorities, due to this increase in knowledge and computation.
By creating a compliance “cyborg” team, a healthcare organization’s privacy experts can work closely with the Protenus AI to examine all spurious behavior. The inclusion of artificial intelligence into the loop does not replace the human expertise. It merely augments it. An interesting facet of machine learning in a cyborg-type application is that not only is the machine helping to augment the capabilities of the human experts, but their knowledge is fed back into the AI system, allowing it to get smarter over time. At its core, machine learning is a collection of mathematical equations, but the more data the system is shown -- for example, by humans resolving alerts as violations or false positives -- the more accurately the system can detect privacy incidents.
Increased efficiency
As the Protenus platform receives more use, it is now capable of scanning hundreds of millions of accesses to patient records every month to detect anomalous activity. This is beyond the reach of even a super human privacy officer. To manually achieve this same goal, a privacy officer would have to review 6,666,667 accesses per day, or 277,777 per hour, or a staggering 77 accesses per second, every single day. Granting a compliance officer five minutes to determine if an access to patient data is suspect would require a health system to employ over 23,000 professionals whose sole focus would be to examine accesses to patient data - all day, every day. However, when working with an AI-powered platform, a small team of compliance officers are capable of going through all these accesses because the AI does the heavy lifting, only presenting the most threatening cases to the organization.
Research on the uses of artificial intelligence have come a long way since the early 1950s. Healthcare leaders have begun to recognize the potential for better protecting patient data when privacy officer expertise is combined with the computation power of artificial intelligence. As health systems grow larger and more complex, it will become imperative to have an extra set of digital eyes reviewing the gigantic volume of accesses to patient data that exists. The power of the cyborg will help healthcare organizations mitigate and ultimately prevent health data breaches that can become costly for the organization and its patients. It’s time for healthcare organizations to embrace the power of the “cyborg” because it will help them combat the common challenges associated with manually detecting health data breaches.
To learn more about what AI can (and can't) do for healthcare security and privacy, join the Privacy FAQ Series for expert insights and actionable advice.
Share this
- November 1, 2024 (1)
- October 1, 2024 (2)
- September 1, 2024 (1)
- August 1, 2024 (1)
- July 1, 2024 (1)
- June 1, 2024 (1)
- May 1, 2024 (1)
- March 1, 2024 (2)
- February 1, 2024 (3)
- January 1, 2024 (1)
- December 1, 2023 (1)
- November 1, 2023 (3)
- October 1, 2023 (3)
- September 1, 2023 (1)
- August 1, 2023 (1)
- July 1, 2023 (2)
- April 1, 2023 (1)
- March 1, 2023 (1)
- February 1, 2023 (1)
- December 1, 2022 (3)
- November 1, 2022 (3)
- October 1, 2022 (1)
- September 1, 2022 (1)
- August 1, 2022 (2)
- June 1, 2022 (4)
- May 1, 2022 (5)
- April 1, 2022 (1)
- March 1, 2022 (4)
- February 1, 2022 (3)
- November 1, 2021 (2)
- October 1, 2021 (3)
- September 1, 2021 (3)
- August 1, 2021 (3)
- July 1, 2021 (4)
- June 1, 2021 (2)
- May 1, 2021 (2)
- April 1, 2021 (2)
- March 1, 2021 (5)
- February 1, 2021 (2)
- January 1, 2021 (1)
- December 1, 2020 (1)
- November 1, 2020 (2)
- October 1, 2020 (2)
- September 1, 2020 (3)
- August 1, 2020 (2)
- July 1, 2020 (2)
- June 1, 2020 (6)
- May 1, 2020 (3)
- April 1, 2020 (4)
- March 1, 2020 (2)
- February 1, 2020 (4)
- January 1, 2020 (2)
- December 1, 2019 (2)
- November 1, 2019 (1)
- October 1, 2019 (1)
- September 1, 2019 (1)
- August 1, 2019 (1)
- June 1, 2019 (1)
- April 1, 2019 (1)
- February 1, 2019 (1)
- January 1, 2019 (1)
- December 1, 2018 (2)
- November 1, 2018 (2)
- October 1, 2018 (2)
- September 1, 2018 (3)
- August 1, 2018 (1)
- July 1, 2018 (2)
- June 1, 2018 (2)
- May 1, 2018 (1)
- April 1, 2018 (1)
- March 1, 2018 (2)
- February 1, 2018 (6)
- January 1, 2018 (2)
- September 1, 2017 (2)
- August 1, 2017 (2)
- June 1, 2017 (2)
- May 1, 2017 (1)
- April 1, 2017 (1)
- March 1, 2017 (2)
- February 1, 2017 (5)
- January 1, 2017 (2)
- December 1, 2016 (3)
- November 1, 2016 (5)
- October 1, 2016 (4)
- September 1, 2016 (8)
- August 1, 2016 (4)
- July 1, 2016 (4)
Subscribe by email
You May Also Like
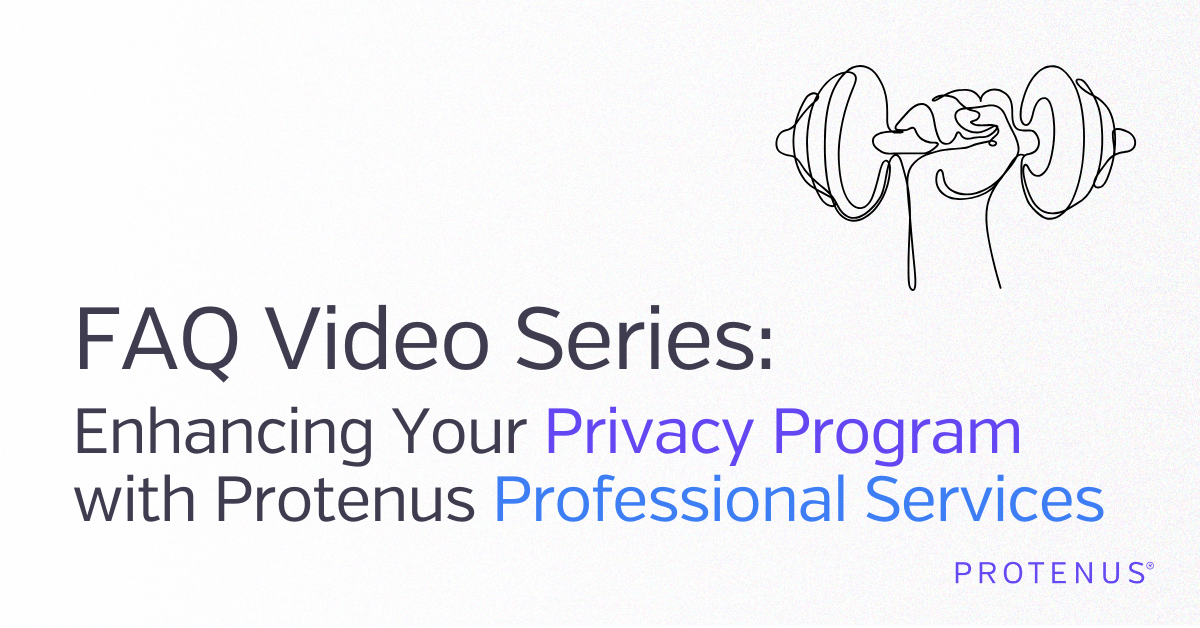
FAQ Video Series: Enhancing Your Privacy Program with Protenus Professional Services
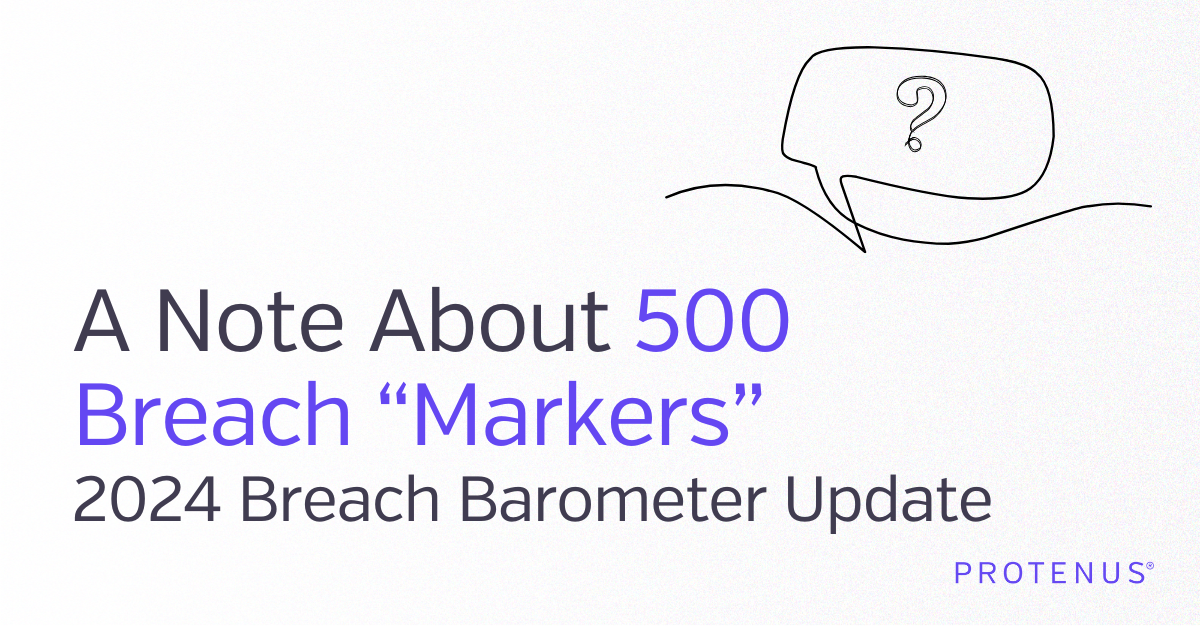
A Note About 500 Breach "Markers"
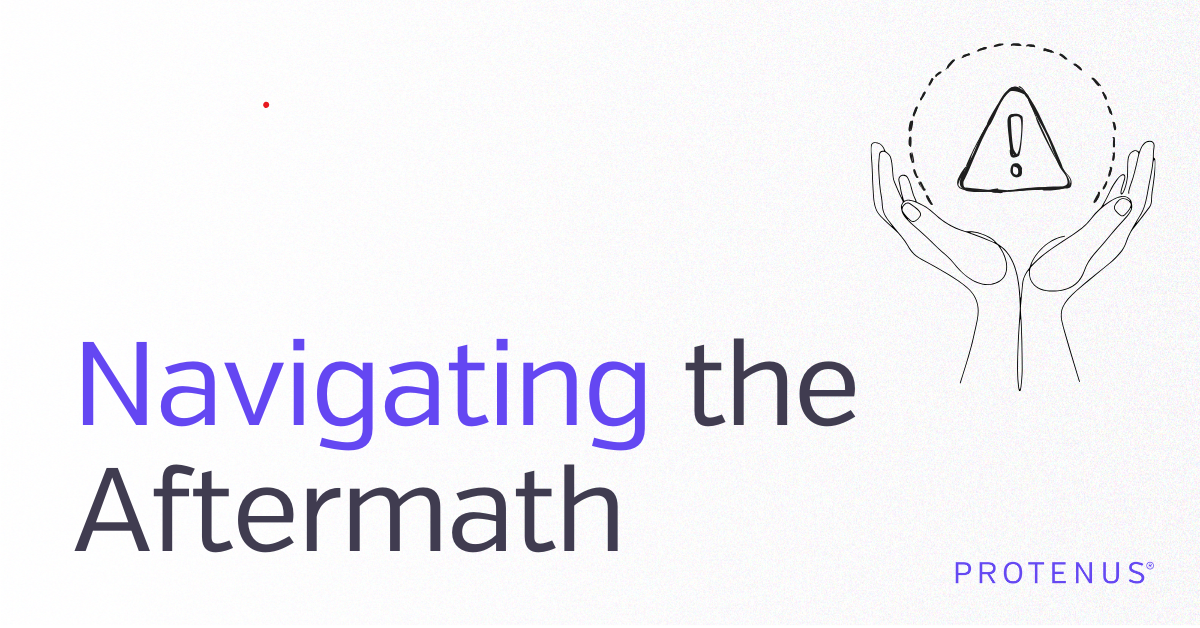