Share this
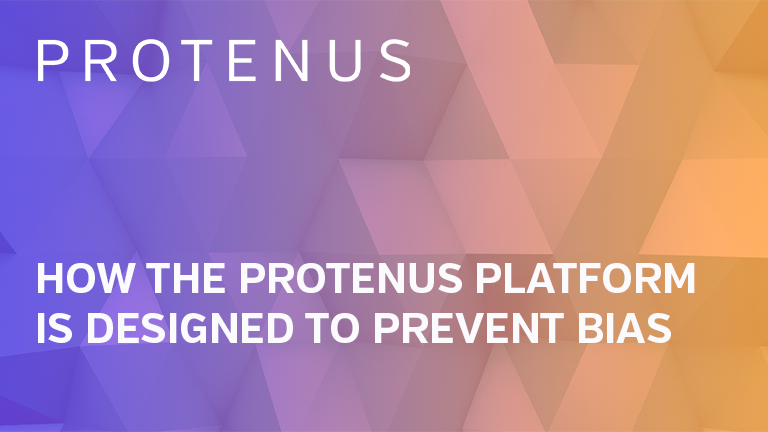
How the Protenus Platform is Designed to Prevent Bias
by Nick Culbertson, CEO and Co-founder, Protenus on July 29, 2020
Right now, as the Protenus team works to act on the ideals of Black Lives Matter, we have found one starting point in the heart of our business: Making sure that our artificial intelligence (AI) does not introduce bias as an unintended consequence of its algorithms.
The need for doing this has been driven home by recent news stories describing how bias in some medical algorithms has had a negative effect on black Americans. That was not the intent of the algorithms or their creators, but it was the result. In one instance, researchers found that an algorithm used to determine whether more than 100 million people needed followup hospital care was racially biased: only 18% of patients directed for followup care were black. The algorithm was built on insurance claims. When it was retrained using patients’ biological data, there was an 82% reduction in bias. In the second instance, a New England Journal of Medicine “Perspective” questioned the practice of race-adjusted practice guidelines, describing how in several medical fields (e.g., cardiology, urology, obstetrics), these guidelines tended to direct resources away from minority patients.
Stories such as these give reason to pause: What do we do to avoid bias in the algorithms we create for Protenus compliance analytics? How can we detect whether bias has been introduced and, if it has, how can we correct it? These are issues Protenus data scientists have begun to consider as we continue to research and develop new products and features.
Building a transparent AI
When we first launched Protenus in 2014, we set out to create an authentic and transparent AI system that our customers could use to readily identify potential compliance violations. Six years later, we have done just that. Our system is ideal for doing what AI does best—gathering vast amounts of sometimes confusing data, processing it, and presenting it for review by humans who are knowledgeable about the subject at hand: In our case, privacy monitoring and drug diversion surveillance.
With our case-based model, Protenus builds a summary outlining a pattern of suspicious behaviors with specific details about which regulations or policies have been violated. Since we do not leverage a black-box approach, there is nothing hidden about how or why a suspicion alert is generated. However, what our customers opt to do with that alert (i.e., decide whether it was a violation, good catch, or false positive) is ultimately up to them. We rely on customer feedback to train our AI to continuously improve its accuracy and ensure that our customers get the most value from the platform.
Avoiding AI bias
The Protenus platform does not collect the kinds of data that often lead to racial bias. We do not collect data about race or ethnicity, and ZIP codes are used only to help match neighbors or shared residences for suspected privacy violations. Instead, our analytics examine user actions and behaviors, rather than demographics, to detect issues violating hospital or regulatory policies.
However, our data scientists have recently begun to consider questions that, until June’s Black Lives Matter protests raised awareness began, had not been top of mind for us.
Among the questions being asked, we’d like to know who is actually targeted when we identify potential cases that are reported to customers? And who is protected? When we report cases to our customers, which ones do they act on and which ones are not acted on? For example, are there identical violations that lead to reprimands for some staff members, or termination of employment for others? By analyzing customer feedback for certain trends about how cases are resolved, we might find ways to advise customers when bias appears to be occurring in response to certain violations. This idea had not come to the fore among our data science team until recent revelations about the potential for bias in AI.
As other data scientists and researchers nationwide reflect on their work, and on new projects, they too should begin to see places where bias could be introduced and amplified. In some instances, data scientists may realize that customers--or bad actors who have customer data--can misuse that data to misconstrue platforms. These questions and strategies for solving them are becoming widespread. Among organizations working to end bias in data and artificial intelligence is Data for Black Lives, which has a network of 4,000 scientists and organizations nationwide aiming to uncover biased algorithms and correcting them—or ridding systems of them.
These are questions many technology companies now face, and will continue to face in the years to come. Until recently, the general public—and even some researchers and scientists—appeared to give little attention to the idea that human biases about race, gender, socioeconomic class and more could influence algorithms that affect our lives, from deciding healthcare treatments to recommending “watch next” on Netflix. The truth is that AI and its algorithms reflect and amplify human bias. It might not be intentional, but it happens.
For those who want to learn more about this issue, I recommend Weapons of Math Destruction. You can read almost any chapter and understand the potential harms of big data when it is used irresponsibly, or with little thought into how it might be misused.
Another good resource for those interested in ethics and data is The Alan Turing Institute, named in honor of the genius whose work cracking the German enigma is credited with saving one million lives in World War II; the Institute includes a research area focused on data ethics in the UK.
“We can only see a short distance ahead, but we can see plenty there that needs to be done.”— Alan Turing
Turing's words are as true today as they were in his lifetime. Ensuring that data science and artificial intelligence are designed for good—and are retrained when bias is found—is an essential part of that work.
Email our team to learn more about how to prevent AI bias within your organization.
Share this
- December 1, 2024 (3)
- November 1, 2024 (1)
- October 1, 2024 (2)
- September 1, 2024 (1)
- August 1, 2024 (1)
- July 1, 2024 (1)
- June 1, 2024 (1)
- May 1, 2024 (1)
- March 1, 2024 (2)
- February 1, 2024 (3)
- January 1, 2024 (1)
- December 1, 2023 (1)
- November 1, 2023 (3)
- October 1, 2023 (3)
- September 1, 2023 (1)
- August 1, 2023 (1)
- July 1, 2023 (2)
- April 1, 2023 (1)
- March 1, 2023 (1)
- February 1, 2023 (1)
- December 1, 2022 (3)
- November 1, 2022 (3)
- October 1, 2022 (1)
- September 1, 2022 (1)
- August 1, 2022 (2)
- June 1, 2022 (4)
- May 1, 2022 (5)
- April 1, 2022 (1)
- March 1, 2022 (4)
- February 1, 2022 (3)
- November 1, 2021 (2)
- October 1, 2021 (3)
- September 1, 2021 (3)
- August 1, 2021 (3)
- July 1, 2021 (4)
- June 1, 2021 (2)
- May 1, 2021 (2)
- April 1, 2021 (2)
- March 1, 2021 (5)
- February 1, 2021 (1)
- January 1, 2021 (1)
- December 1, 2020 (1)
- November 1, 2020 (2)
- October 1, 2020 (2)
- September 1, 2020 (3)
- August 1, 2020 (2)
- July 1, 2020 (2)
- June 1, 2020 (6)
- May 1, 2020 (3)
- April 1, 2020 (4)
- March 1, 2020 (2)
- February 1, 2020 (4)
- January 1, 2020 (2)
- December 1, 2019 (2)
- November 1, 2019 (1)
- October 1, 2019 (1)
- September 1, 2019 (1)
- August 1, 2019 (1)
- June 1, 2019 (1)
- April 1, 2019 (1)
- February 1, 2019 (1)
- January 1, 2019 (1)
- December 1, 2018 (2)
- November 1, 2018 (2)
- October 1, 2018 (2)
- September 1, 2018 (3)
- August 1, 2018 (1)
- July 1, 2018 (2)
- June 1, 2018 (2)
- May 1, 2018 (1)
- April 1, 2018 (1)
- March 1, 2018 (2)
- February 1, 2018 (6)
- January 1, 2018 (2)
- September 1, 2017 (2)
- August 1, 2017 (2)
- June 1, 2017 (2)
- May 1, 2017 (1)
- April 1, 2017 (1)
- March 1, 2017 (2)
- February 1, 2017 (5)
- January 1, 2017 (2)
- December 1, 2016 (3)
- November 1, 2016 (5)
- October 1, 2016 (4)
- September 1, 2016 (8)
- August 1, 2016 (4)
- July 1, 2016 (4)
Subscribe by email
You May Also Like
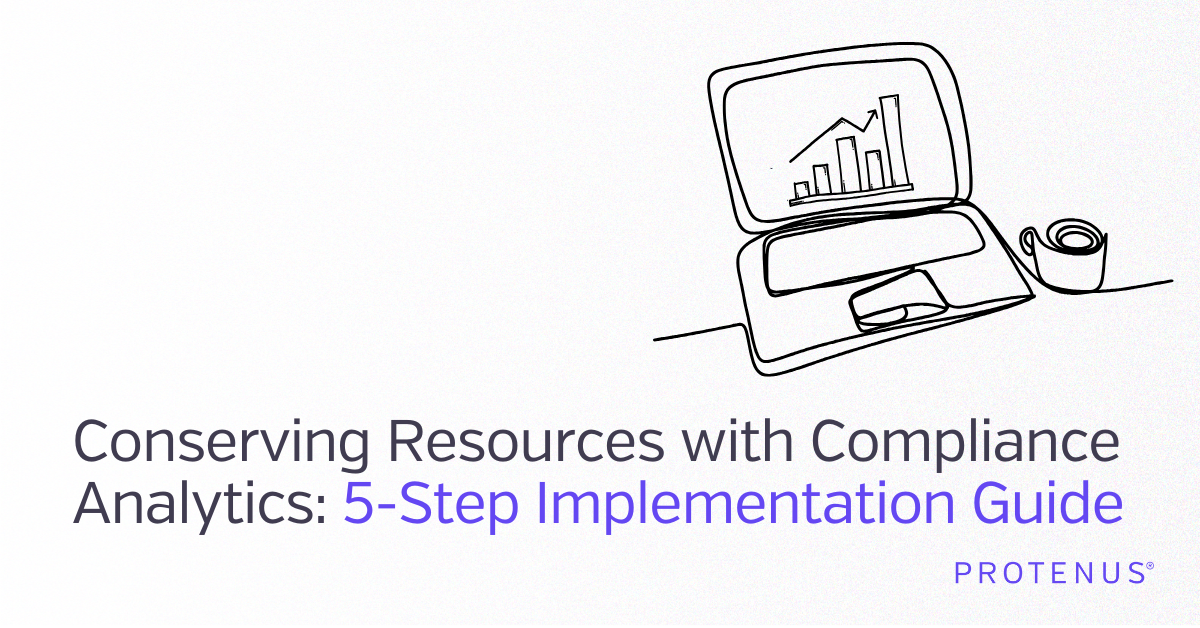
Conserving Resources with Compliance Analytics: A 5-Step Implementation Guide
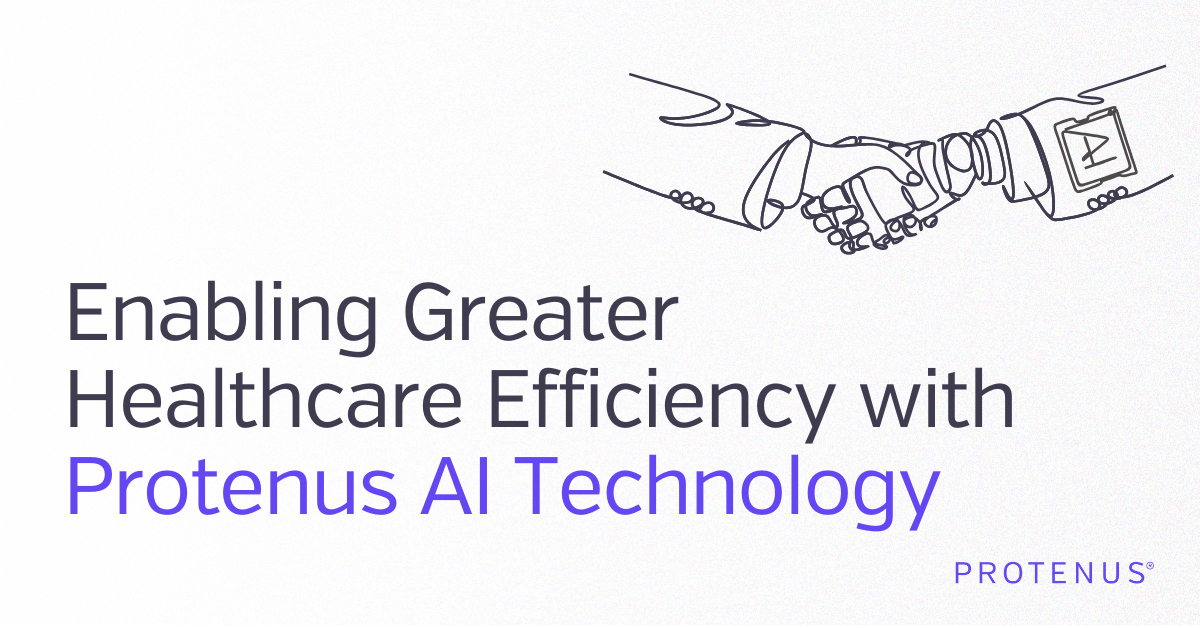
Enabling Greater Healthcare Efficiency with Protenus AI Technology
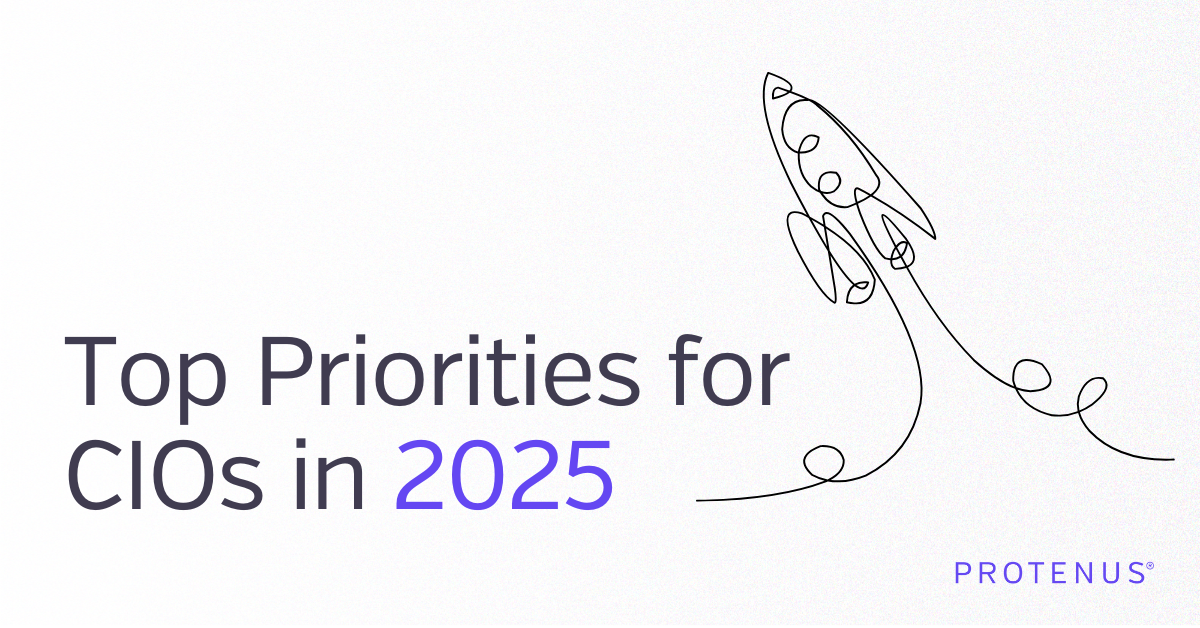